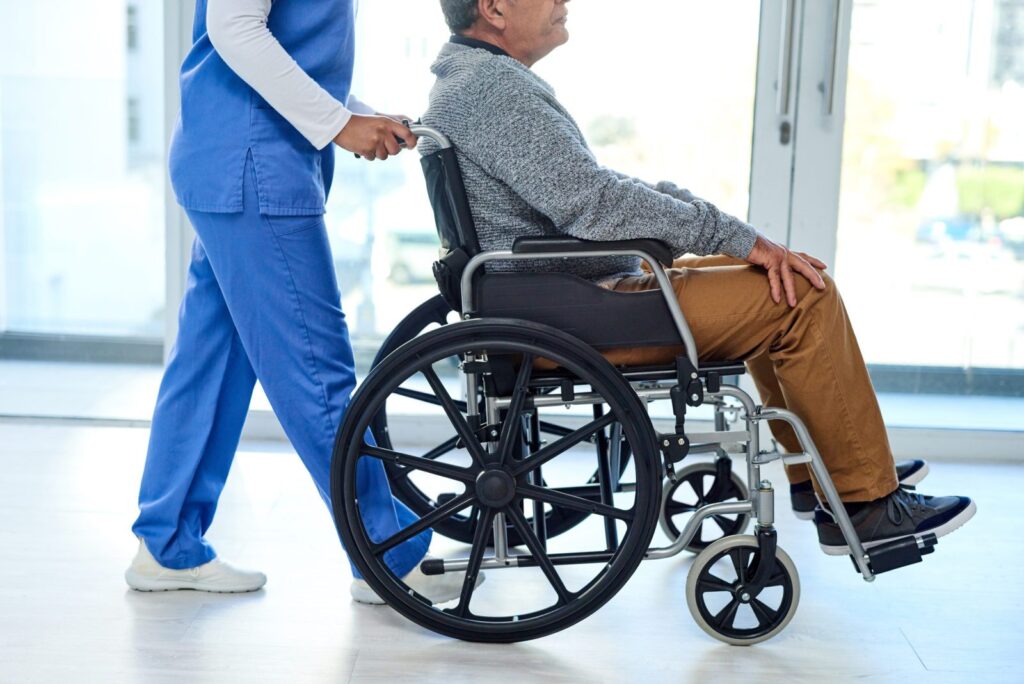
How can predictive modeling help support patients through transitions and ensure they continue to receive the care they need, adhere to medication regimens, avoid readmissions and become more actively involved in their own care?
By Medecision
Transitions are an essential, unavoidable and often difficult part of any journey. This is especially true when it comes to the patient healthcare journey. Far too often, when patients are discharged from a hospital, they end up being readmitted shortly after their hospital stay. According to the latest statistics from the Agency for Healthcare Research and Quality, there were 3.8 million adult hospital readmissions within 30 days in 2018, with an average readmission cost of $15,200,. Taking these averages into consideration, it’s no wonder that readmissions cost the U.S. healthcare system billions annually, or that a majority of hospitals are grappling with readmission penalties.
The process of admitting, discharging and transferring patients between units and across facilities is routine for most hospitals and clinicians. But how can they ensure these handoffs are executed efficiently and effectively as patients move from one care setting to another? This is where fully integrated data analytics and predictive modeling can make a powerful difference. Implementing these tools can help improve the continuity of care by reducing readmissions and equipping providers with the insight they need to deliver the right care at the right time and make the best decisions for each individual patient.
Take UnityPoint Health in West Des Moines, Iowa, for example. Using an integrated predictive analytics approach, a hospital within the regional health network improved its risk-adjusted readmissions indexes by 40% over three years, surpassing its own internal performance targets and becoming a top performer in the health system.
Predictive modeling must be integrated across the continuum of care to achieve this kind of success. In many organizations, these models are isolated—applied to certain silos but not shared across departments or integrated throughout systemwide workflows.
How Predictive Modeling Helps Transitional Care
So exactly how can predictive modeling ease transitions for hospitals? Here are three significant ways .
- Engage at-risk patients while reducing costs. “Predictive models empower [hospitals] to find moderate-risk individuals—both patients and prospects—before they become high-risk,” according to a 2017 whitepaper from Healthgrades. Identifying patients before they become high risk can lower healthcare costs in the long term.
- Determine mortality rates of patients in the intensive care unit. Predictive analytics can be used to “help doctors make decisions regarding what resources to allocate to individual patients,” according to a 2021 article in HealthIT Analytics. Using data and algorithms, clinicians are better able to predict the mortality of patients in the intensive care unit and help clinicians make treatment decisions, according to research published in the Annals of Intensive Care.
- Streamline patient flow in the emergency department. Crowding in the emergency department can lead to long wait times and delays in diagnosis and treatment. Predictive tools that enable clinicians and care coordinators to visually understand patient acuity trends in real time can help them prioritize and improve patient flow and identify the most at-risk patients so they can move them to a higher level of care faster. As a result, hospitals and healthcare systems can serve more patients, according to a report from Philips.
Additionally, after a patient leaves the hospital, predictive modeling can help guide decisions about the next stop in the journey: whether it be at-home care, a skilled nursing facility or another care setting. Care managers can use analytics tools to support patients through these transitions and ensure they continue to receive the care they need, adhere to medication regimens, avoid readmissions and become more actively involved in their own care.
Developing a Predictive Modeling Strategy
If your organization is in the early stages of developing or adopting predictive models for improving care and reducing readmissions, there are a few factors to keep in mind. First and foremost, it should be a multidisciplinary effort that incorporates input from everyone connected to patients, from primary care doctors, nurses and care managers to home health and skilled nursing facility workers. Also, to develop useful predictive models for all care teams, it’s important to fully understand the readmissions journey for patients and the factors that may have influenced their return to the hospital, such as caregiver concerns, medication issues or whether they attended follow-up appointments.
Hospitals can draw insight from other patient data as well, including healthcare utilization, diagnosis history, social determinants of health and details about patient visits, including vitals, labs and medications.
Identifying the necessary data for a predictive model is only half the battle—to make it actionable, it requires certain capabilities, such as:
- Scorecards and dashboards with key performance indicators and standardized, ongoing reports that can be easily accessed and understood
- Exploratory analysis with the ability to answer unanticipated questions and execute exploratory versus standard reporting without data dumping
- Predictive modeling that utilizes advanced analytics and statistical methodologies
To best leverage predictive models, it also helps to know where in the patient journey to implement them and to be able to customize the data to show relevant views for different care teams, service lines, diagnoses groups, etc.
Identifying the Right Data
One of the biggest challenges of implementing predictive modeling into a readmissions reduction strategy is pinpointing the data needed to make a meaningful impact. Not sure where to start? Take these pointers from hospitals that have successfully used predictive analytics tools to reduce readmissions, as reported by Managed Healthcare Executive.
Ask the right questions. To tackle readmission rates at UnityPoint Health, nurses started asking patients “Why do you think you’re back?” after they were readmitted within 30 days. This encouraged patients to speak more openly about challenges they faced such as lack of access to follow-up appointments, food insecurity and the inability to pay for medications. The hospital combined these patient narratives with retrospective data on readmissions to create a readmissions risk score for each patient, which care teams can view via a color-coded dashboard. They use these scores to plan interventions for high-risk patients and keep follow-up appointment slots open for them.
Look for trends. When the University of Kansas Health system discovered that patients with diabetes were more likely than other patients to be readmitted three times within 90 days, the health system decided to use predictive analytics to dig deeper. It found that chronic conditions, inability to access follow-up care and patient discharge disposition (having a family member in the home or staying in a skilled nursing or rehab facility) were the biggest drivers of readmissions for diabetes patients. With this insight, hospital leaders developed interventions for patients, such as connecting them with a case manager to help with appointments and education, and reduced readmissions in that population by 11%.
Follow the money. Saint Augustine, Florida-based Flagler Hospital used predictive analytics to reduce variation in care for the hospital’s pneumonia patients. Analyzing patient treatment over four years, the hospital found that pneumonia patients were getting daily X-rays and blood tests without improvement in outcomes. However, when they were given nebulizer treatments early on in their hospital stay, their outcomes improved. By making nebulizer treatment standard for newly admitted pneumonia patients, the hospital was able to shorten their length of stay by two days, reduce readmissions, and cut down on testing and the cost of care, saving thousands of dollars per patient.
Adopting fully integrated predictive modeling into your workflows can be a game-changer for easing care transitions for at-risk patients and developing proactive interventions to keep them healthy and out of the hospital. Not only will it make the healthcare journey smoother for patients, but it will also make delivering high-quality, affordable care easier for your organization.
About The Author: Medecision
Medecision is a leader in cloud-based, data-powered healthcare solutions, enabling risk-bearing entities to achieve improved health outcomes, optimized medical costs, reduced administrative costs, and personalized engagement. Our Aerial platform supports over 10% of the U.S. population, marking a significant milestone in advancing healthcare efficiency and effectiveness.
More posts by Medecision